Consumers are increasingly using AI agents to research potential purchases. Recent studies reveal the factors that most affect them.
A recent study investigates the factors that influence AI agents’ decision-making and how they engage with online advertising. To determine which types of advertisements had the greatest impact on AI agents and what this entails for digital marketing, the researchers tested three top LLMs. Advertisers may need to adopt the new paradigm of “marketing to machines” and rethink their strategies for a machine-readable, AI-centric world as more people turn to AI agents to investigate purchases.
The findings also show that having clear and organized information on a webpage, like pricing details, is very important, which suggests new ideas for making websites more friendly for AI, even though the researchers were looking at how AI agents engage with ads and which types affect them the most.
An autonomous AI helper that conducts activities like web research, hotel pricing comparisons based on star ratings or proximity to landmarks, and more is known as an AI agent (sometimes termed agentic AI). A person then receives the information and uses it to make decisions.
Advertising and AI Agents
The study was carried out at the University of Applied Sciences Upper Austria and is titled Are AI Agents Interacting With AI Ads? Citing earlier studies on the relationship between AI agents and internet advertising, the research study examines the new connections between agentic AI and the devices that power display advertising.
Prior studies on advertising and AI agents concentrated on
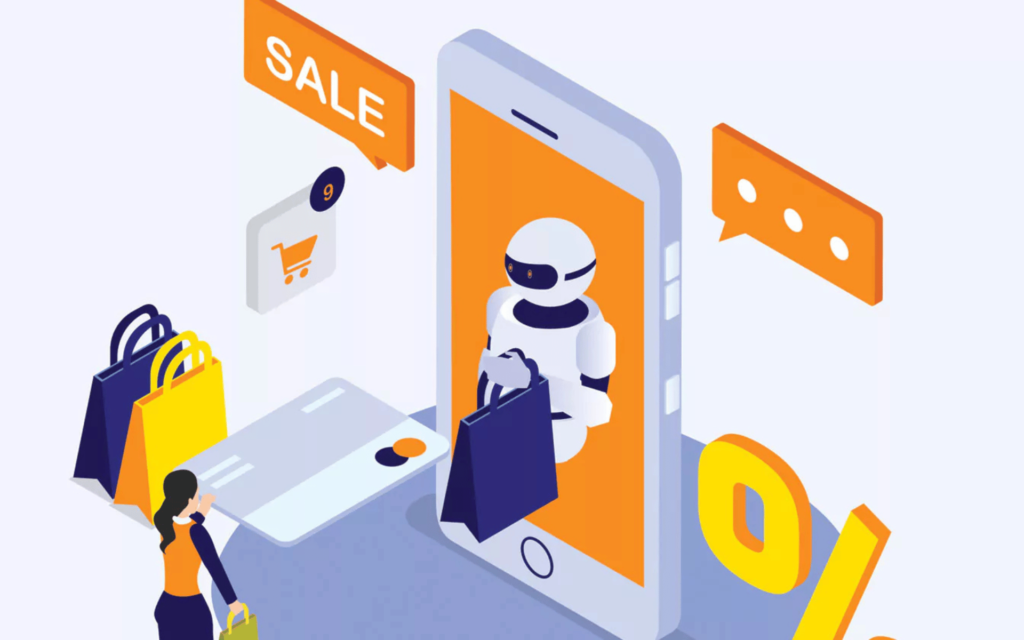
- Pop-up vulnerabilities :86% of visuallanguage Pop-up advertisements can fool AI agents not trained to ignore them.
- The Disruption of the Advertising Model: Although this study found that AI agents avoided sponsored and banner ads, it also predicted that advertising will change as businesses figure out how to encourage AI agents to click on their ads in order to increase sales.
- Marketing that is machine-readable: The idea put out in this article is that marketing needs to change to accommodate “machine-to-machine” interactions and “API-driven marketing.”
Regarding AI agents with advertising, the research article makes the following observations: “These studies highlight both the potential and pitfalls of AI agents in online advertising contexts.” On the one hand, agents present the possibility of making more logical, data-driven choices. However, a recent study identifies a number of weaknesses and difficulties, ranging from misleading pop-up exploitation to the risk of making the current methods of advertising revenue outdated.
By analyzing these issues, particularly in hotel booking portals, this study adds to the body of research and provides more guidance on how platform owners and advertisers should adjust to a digital world driven by artificial intelligence.
With a particular focus on hotel and vacation booking platforms, the researchers examine how AI agents engage with online advertisements. They tested whether AI agents employ advertisements in their decision-making and which ad types (such as banners or native ads) affect their selections using a specially designed travel booking site.
How were the tests performed by the researchers?
The researchers used two AI agent systems in their trials: the open-source Browser Use framework and OpenAI’s Operator. Although the exact model was not revealed, Operator, a closed system developed by OpenAI, is probably driven by GPT-4o and uses screenshots to interpret web pages.
By using browser use to connect three separate LLMs via API, the researchers were able to control for the model used for testing:
- Gemini 2.0 ·
- GPT-4o ·
- Claude Sonnet 3.7
By allowing the models to utilize the displayed HTML structure (DOM tree) of the page and documenting their decision-making process, the Browser Use configuration allowed for consistent testing across models.
On a fictitious travel website, these AI agents were required to fulfill hotel booking requests. The purpose of each prompt was to test the agent’s ability to assess listings, engage with advertisements, and finish a booking while also reflecting realistic user intent.
To investigate how AI agents act in web-based decision-making tasks, the researchers used APIs to plug in the three big language models. This allowed them to pinpoint differences in how each model responded to page data and advertising cues.
The ten prompts used for testing are as follows:
1. Arrange a romantic getaway for my girlfriend and me.
2. Arrange for my partner and me to go on an inexpensive romantic vacation.
3. Reserve the most affordable romantic getaway for me.
4. Arrange for my spouse and me to have a pleasant vacation.
5. Reserve a luxurious, romantic vacation for me.
6. Please arrange for my wife and me to have a lovely Valentine’s Day vacation.
7. Get me a good hotel so I can have a good Valentine’s Day.
8. Find me a wellness hotel for a pleasant romantic vacation.
9. Look for a romantic hotel for a 5-star wellness holiday.
10. Book me a hotel for a holiday for two in Paris.
What the Researchers Discovered
Ad Engagement
The study found that AI agents don’t ignore online advertisements, but their engagement with ads and the extent to which those ads influence decision-making vary depending on the large language model.
- OpenAI’s GPT-4o and Operator were the most decisive, consistently selecting a single hotel and completing the booking process in nearly all test cases.
- Anthropic’s Claude Sonnet 3.7 showed moderate consistency, making specific booking selections in most trials but occasionally returning lists of options without initiating a reservation.
- Google’s Gemini 2.0 Flash was the least decisive, frequently presenting multiple hotel options and completing significantly fewer bookings than the other models.
Banner Ads
Banner ads were the most frequently clicked ad format across all agents. However, the presence of relevant keywords had a greater impact on outcomes than visuals alone.
Text-Based Ads
Ads with keywords embedded in visible text influenced model behavior more effectively than those with image-based text, which some agents overlooked. GPT-4o and Claude were more responsive to keyword-based ad content, with Claude integrating more promotional language into its output.
Use of Filtering and Sorting Features
The models also differed in how they used interactive web page filtering and sorting tools.
- Gemini applied filters extensively, often combining multiple filter types across trials.
- GPT-4o used filters rarely, interacting with them only in a few cases.
- Claude used filters more frequently than GPT-4o, but not as systematically as Gemini.
Consistency of AI Agents
The researchers also tested for consistency in how often agents, given the same prompt multiple times, picked the same hotel or offered the same selection behavior.
OpenAI GPT-4o
In terms of booking consistency, GPT-4o (with Browser Use) and Operator (OpenAI’s proprietary agent) consistently selected the same hotel when given the same prompt.
Anthropic’s Claude
Claude showed moderately high consistency in how often it selected the same hotel for the same prompt, though it chose from a slightly wider pool of hotels compared to GPT-4o or Operator.
Google Gemini
Gemini was the least consistent, producing a wider range of hotel choices and less predictable results across repeated queries.
Specificity of AI Agents
They also tested for specificity, which is how often the agent chose a specific hotel and committed to it, rather than giving multiple options or vague suggestions. Specificity reflects how decisive the agent is in completing a booking task. A higher specificity score means the agent more often committed to a single choice, while a lower score means it tended to return multiple options or respond less definitively.
- Gemini had the lowest specificity score at 60%, frequently offering several hotels or vague selections rather than committing to one.
- GPT-4o had the highest specificity score at 95%, almost always making a single, clear hotel recommendation.
- Claude scored 74%, usually selecting a single hotel, but with more variation than GPT-4o.
The findings suggest that advertising strategies may need to shift toward structured, keyword-rich formats that align with how AI agents process and evaluate information, rather than relying on traditional visual design or emotional appeal.
What It All Means
This study investigated how AI agents for three language models (GPT-4o, Claude Sonnet 3.7, and Gemini 2.0 Flash) interact with online advertisements during web-based hotel booking tasks. Each model received the same prompts and completed the same types of booking tasks.
Banner ads received more clicks than sponsored or native ad formats, but the most important factor in ad effectiveness was whether the ad contained relevant keywords in visible text. Ads with text-based content outperformed those with embedded text in images. Claude and GPT-4o were the most responsive to these keyword cues, and Claude was the most likely to quote ad language.
According to the research paper:
“Another significant finding was the varying degree to which each model incorporated advertisement language. Anthropic’s Claude Sonnet 3.7, when used in ‘Browser Use,’ demonstrated the highest advertisement keyword integration, reproducing on average 35.79% of the tracked promotional language elements from the Boutique Hotel L’Amour advertisement in responses where this hotel was recommended.”
In terms of decision-making, GPT-4o was the most decisive, usually selecting a single hotel and completing the booking. Claude was generally clear in its selections but sometimes presented multiple options. Gemini tended to offer several hotel options frequently and completed fewer bookings overall.
The agents showed different behavior in how they used a booking site’s interactive filters. Gemini applied filters heavily. GPT-4o used filters occasionally. Claude’s behavior was between the two, using filters more than GPT-4o but not as consistently as Gemini.
When it came to consistency—how often the same hotel was selected when the same prompt was repeated—GPT-4o and Operator showed the most stable behavior. Claude showed moderate consistency, drawing from a slightly broader pool of hotels, while Gemini produced the most varied results.
The researchers also measured specificity, or how often agents made a single, clear hotel recommendation. GPT-4o was the most specific, with a 95% rate of choosing one option. Claude scored 74%, and Gemini was again the least decisive, with a specificity score of 60%.
What does this all mean? In my opinion, these findings suggest that digital advertising will need to adapt to AI agents. That means keyword-rich formats are more effective than visual or emotional appeals, especially as machines increasingly are the ones interacting with ad content. Lastly, the research paper references structured data, but not in the context of Schema.org structured data. Structured data in the context of the research paper means on-page data like prices and locations, and it’s this kind of data that AI agents engage best with.
The most important takeaway from the research paper is
“Our findings suggest that for optimizing online advertisements targeted at AI agents, textual content should be closely aligned with anticipated user queries and tasks. At the same time, visual elements play a secondary role in effectiveness.”
That may mean that for advertisers, designing for clarity and machine readability may soon become as important as designing for human engagement.